Reinforcement technology is constantly evolving, capturing the imagination of both industries and researchers eager to harness its potential. The myriad of ties in reinforcement learning are pivotal to understanding its vast applications, leading to increased efficiency and innovation in fields ranging from robotics to digital marketing.
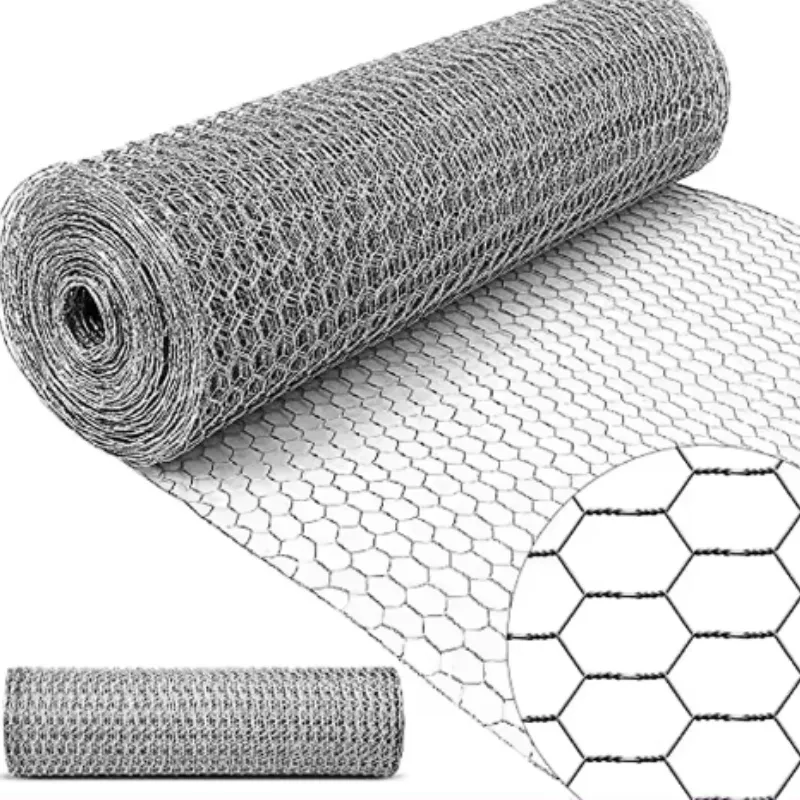
The foundation of reinforcement learning lies in its ability to adapt through interactions with the environment. The ties or types of connections formed during these interactions are crucial for crafting specific learning strategies. Among the primary ties in reinforcement learning, there are three significant types positive ties, negative ties, and neutral ties.
Positive ties are the backbone of motivational force in a learning agent. These ties are formed when actions are followed by rewarding outcomes. For instance, in a robotic stock-picking system, positive ties might be formed when the chosen stock appreciates in value. The system then records and reinforces this action-environment connection, encouraging future similar actions. This process not only enhances performance efficiency but also accelerates the learning curve, allowing more strategic decisions over time.
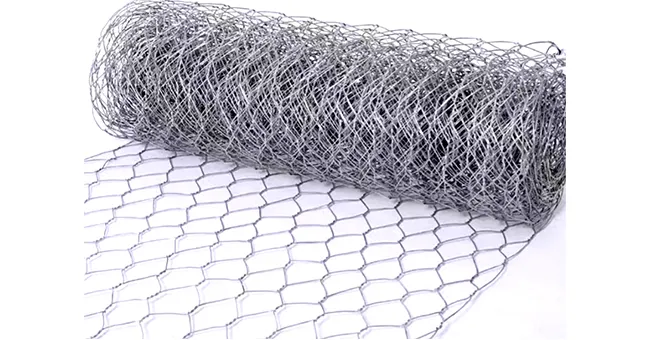
Negative ties, on the other hand, arise when actions lead to undesirable outcomes. They serve as critical learning points, ensuring the agent avoids similar actions in the future. Continuing with the stock-picking example, negative ties would develop when the system invests in stocks that subsequently plummet. While initially perceived as setbacks, these ties are instrumental in refining the decision-making process. By assessing what actions led to poor performance, the system gradually optimizes its strategy to minimize risks.
types of ties in reinforcement
Between the extremes of positive and negative ties lies the domain of neutral ties. These connections occur when actions have no significant positive or negative outcome. Understanding the role of neutral ties is essential, especially in complex environments where immediate consequences are not obvious. For an online retail platform using reinforcement learning for personalized marketing, neutral ties might occur when certain product recommendations neither increase nor decrease sales. Identifying these neutral instances helps in recognizing patterns that require further exploration or adjustment.
The integration of these ties makes reinforcement learning robust and adaptive. The ability to classify experiences and convert them into actionable knowledge is a testimony to the sophisticated algorithms driving this technology. However, the successful application of reinforcement learning requires expertise and trustworthiness. Stakeholders must ensure that the data guiding the learning processes is accurate and that the outcomes are ethical and fair. Ensuring data privacy and transparency builds trust, reinforcing the system's authority and credibility.
In terms of practical application, experts often engage in continuous monitoring and tuning of these ties. They leverage simulations and pilot tests to refine strategies in safe environments before real-world application. This expertise assures that reinforcement learning systems are not only theoretically sound but also practically viable.
In summary, the various types of ties in reinforcement learning—positive, negative, and neutral—offer a nuanced framework for developing intelligent, self-improving systems. Each tie provides valuable insights, contributing to a comprehensive learning strategy. As industries increasingly rely on these dynamic systems, the need for expert-led implementation becomes paramount, cementing the technology's place as both authoritative and trustworthy in today's digital landscape.